The Cohort Study Power Calculator is a tool designed to estimate the statistical power of a cohort study. Statistical power represents the probability of detecting a true effect or association in a study, assuming it exists. A power calculator helps researchers ensure their study is sufficiently designed to detect meaningful differences between groups, thereby minimizing the risk of Type II errors (failing to detect an effect). This calculator is essential in planning epidemiological and clinical studies, where accurate sample size determination and effect size estimation are critical for reliable results. It belongs to the category of statistical and epidemiological tools.
Formula of Cohort Study Power Calculator
The power of a cohort study is calculated using the formula:
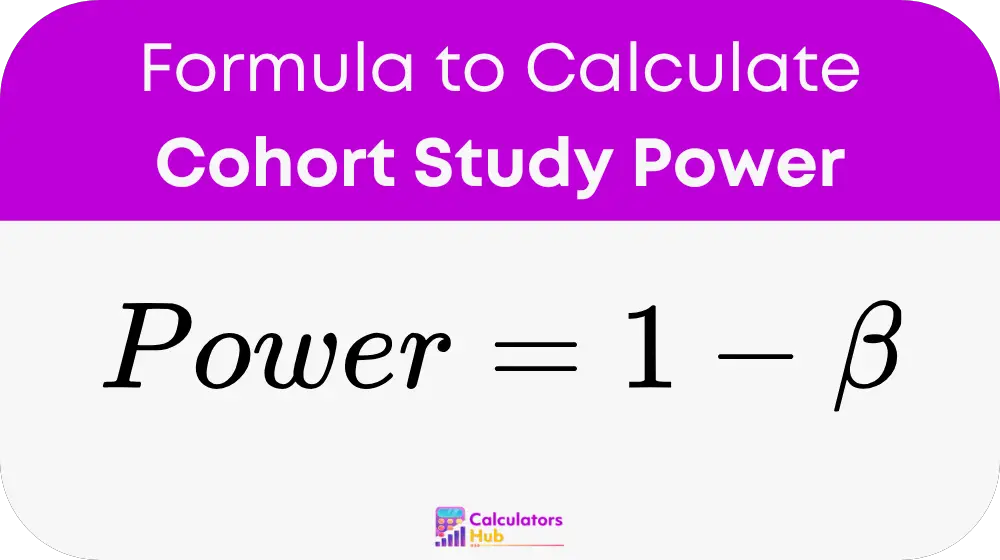
Where:
- β is the Type II error rate (the probability of failing to detect a true effect).
The power depends on various factors, including sample size, effect size, significance level, and variability in the outcome. The detailed formula for power calculation in a cohort study is:
Power = Φ[(Zα + Zβ) – √(n × p1 × (1 – p1) + n × p2 × (1 – p2)) / |p1 – p2|]
Detailed Formulas for Variables:
Zα (Critical Value for Significance Level):
Zα = Φ⁻¹(1 – α/2)
Where:
- α is the significance level (e.g., 0.05 for 95% confidence).
Zβ (Critical Value for Power):
Zβ = Φ⁻¹(1 – Power)
Variance Terms:
- Variance for the exposed group: n × p1 × (1 – p1)
- Variance for the unexposed group: n × p2 × (1 – p2)
Effect Size:
Effect Size = |p1 – p2|
This is the absolute difference in proportions between the exposed and unexposed groups.
Sample Size (n):
Adjustments can be made for unequal group sizes.
Pre-Calculated Table for Power Calculations
The table below provides an overview of pre-calculated power for common scenarios in cohort studies. This table can be helpful for quick reference:
Significance Level (α) | Power (1 – β) | Sample Size per Group (n) | Effect Size (p1 – p2) |
---|---|---|---|
0.05 | 0.80 | 50 | 0.10 |
0.05 | 0.90 | 100 | 0.15 |
0.01 | 0.80 | 150 | 0.20 |
0.01 | 0.95 | 200 | 0.25 |
This table simplifies power estimations for commonly encountered study designs.
Example of Cohort Study Power Calculator
Suppose a cohort study is designed to evaluate the effect of exposure to a risk factor on a specific outcome. The following data are provided:
- Proportion of outcome in the exposed group (p1) = 0.30.
- Proportion of outcome in the unexposed group (p2) = 0.20.
- Sample size per group (n) = 100.
- Significance level (α) = 0.05.
Step 1: Calculate Effect Size
Effect Size = |p1 – p2| = |0.30 – 0.20| = 0.10
Step 2: Calculate Variance Terms
- Variance for the exposed group = n × p1 × (1 – p1) = 100 × 0.30 × (1 – 0.30) = 21.00
- Variance for the unexposed group = n × p2 × (1 – p2) = 100 × 0.20 × (1 – 0.20) = 16.00
Step 3: Calculate Z-scores
Zα = Φ⁻¹(1 – α/2) = Φ⁻¹(0.975) ≈ 1.96
Zβ = Φ⁻¹(1 – Power) (assume desired Power = 0.80) ≈ 0.84.
Step 4: Calculate Power
Substitute values into the power formula:
Power = Φ[(1.96 + 0.84) – √(21 + 16) / 0.10]
After simplifying:
Power ≈ 0.80 (or 80%)
This result indicates that the study has an 80% chance of detecting the true effect.
Most Common FAQs
Power ensures that a study has a high probability of detecting a meaningful effect, reducing the risk of Type II errors and increasing the reliability of results.
Key factors include sample size, effect size, significance level (α), and variability in the outcome of interest.
Yes, adjustments should be made for unequal group sizes to ensure accurate power estimation. Most calculators accommodate these adjustments.