Multiple linear regression involves more than one independent variable to predict a dependent variable, unlike simple linear regression, which uses only one. Here, the R² value plays a crucial role by quantifying how well the combination of variables explains the variance observed.
Formula of Multiple Coefficient of Determination Calculator
To calculate R² in multiple regression scenarios, use the following formula:
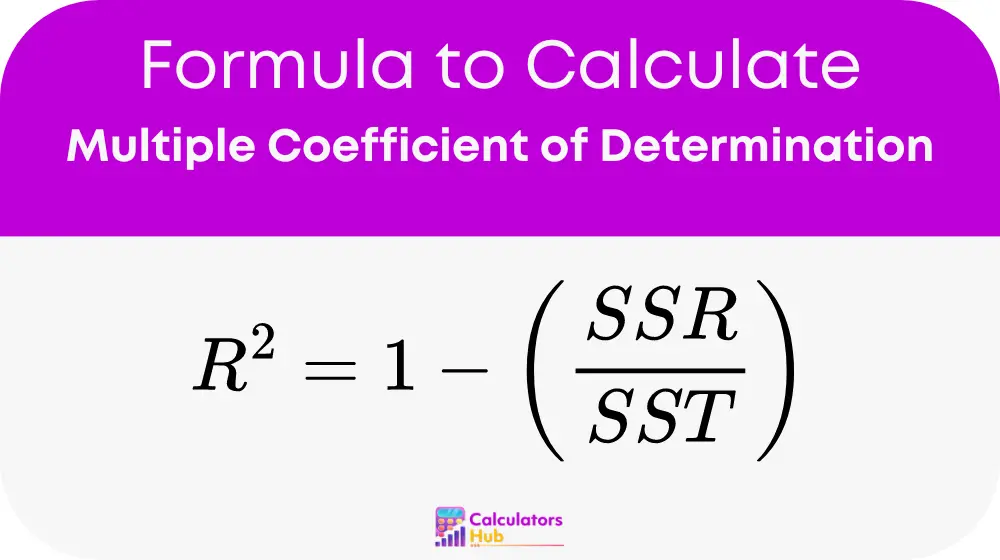
- R²: This is the coefficient of multiple determination, ranging between 0 and 1. A higher R² indicates a better model fit.
- SSR (Sum of Squared Residuals): Represents the variation in the dependent variable that is not explained by the model.
- SST (Total Sum of Squares): Denotes the total variance in the dependent variable.
Understanding each component is crucial as they collectively determine the effectiveness of your regression model in explaining the data.
Relevant Calculations and Conversions
It is helpful to have a reference for related calculations that frequently accompany the use of R². Below is a table summarizing these terms and their significance:
Term | Description | Calculation Needed |
---|---|---|
SSR | Sum of Squared Residuals | Sum of squared differences between predicted and actual values |
SST | Total Sum of Squares | Sum of squared differences between actual values and their mean |
Adjusted R² | Modified R² accounting for number of predictors | AdjustedR2=1−[(1−R2)(n−1)/(n−p−1)] |
These metrics are integral to a comprehensive understanding of your regression model's predictive power and accuracy.
Example of Multiple Coefficient of Determination Calculator
Consider a dataset with multiple predictors. Here’s how you would calculate R²:
- Compute the mean of the dependent variable.
- Calculate SST and SSR using their respective formulas.
- Plug these values into the R² formula.
This process will yield your R² value, indicating the proportion of the dependent variable's variance explained by the model. Interpreting this value is critical: a higher R² suggests a better model fit, but it is essential to consider the potential for overfitting.
Most Common FAQs
An R² value near 1 indicates that the model explains a large portion of the variance in the dependent variable using the independent variables included. This measurement helps assess the quality and effectiveness of a model.
R² values are beneficial for comparing the performance of different models. By evaluating the R², analysts can select a model that appropriately balances complexity and accuracy.
Not necessarily; a very high R² might indicate that the model is overly complex, potentially overfitting the data. It's crucial to balance the model's complexity with its predictive power and ensure it generalizes well to other data sets.